- The AI Timeline
- Posts
- The AI Timeline #3 - Make Tik-Tik videos from Images
The AI Timeline #3 - Make Tik-Tik videos from Images
Latest AI Research Explained Simply
Research Papers x 3🕺 Animate Anyone 🌟 Qwen-72B 💻 Extracting Training Data from ChatGPT | Industry News x 3📽️ Pika 1.0 💎 GNoME 🚄 SDXL Turbo |
Research Papers
🕺 Animate Anyone: Consistent and Controllable Image-to-Video Synthesis for Character Animation
Li Hu, Xin Gao, Peng Zhang, Ke Sun, Bang Zhang, Liefeng Bo

Overview:
Inspiration: Modified AnimateDiff architecture enabling animation of a character from a single image, driven by a sequence of poses

Animate Anyone Model Architecture
Architecture
ReferenceNet: merges detailed features via spatial attention which enables highly consistent regeneration of appearance features from a reference image

Ours = ReferenceNet
Pose Encoding: Adoption of a lightweight ControlNet-based “Pose Guider” for pose encoding to direct character's movements and employ an effective temporal modeling approach to ensure smooth inter-frame transitions between video frames

pose-driven zero-shot character animation
High-Fidelity Representation: Utilization of CLIP encoding for the reference image, enhancing fidelity via cross-attention in a denoising U-Net. The auxiliary ReferenceNet further ensures detailed input image representation
Training Methodology
Stage 1: Spatial training focusing on frame reconstruction, using SD checkpoint-initialized denoising U-Net and ReferenceNet, with a Gaussian-initialized Pose Guider
Stage 2: Isolated training of temporal attention, in line with AnimateDiff norms
Evaluation
Result: animate both real life and arbitrary characters with extremey consistency, and utilizes inpainting for background and subject coherence
FPS: Remarkable coherence in longer animations exceeding 24 frames
Author’s Notes: Easily state of the art performance with subject consistency and video quality I’ve never seen before

comparison with previous research
🌟 通义千问-72B(Qwen-72B)
Jinze Bai, Shuai Bai, Yunfei Chu, Zeyu Cui, Kai Dang, Xiaodong Deng, Yang Fan, Wenbin Ge, Yu Han, Fei Huang, Binyuan Hui, Luo Ji, Mei Li, Junyang Lin, Runji Lin, Dayiheng Liu, Gao Liu, Chengqiang Lu, Keming Lu, Jianxin Ma, Rui Men, Xingzhang Ren, Xuancheng Ren, Chuanqi Tan, Sinan Tan, Jianhong Tu, Peng Wang, Shijie Wang, Wei Wang, Shengguang Wu, Benfeng Xu, Jin Xu, An Yang, Hao Yang, Jian Yang, Shusheng Yang, Yang Yao, Bowen Yu, Hongyi Yuan, Zheng Yuan, Jianwei Zhang, Xingxuan Zhang, Yichang Zhang, Zhenru Zhang, Chang Zhou, Jingren Zhou, Xiaohuan Zhou, Tianhang Zhu

Qwen-72B Model Evaluation
Overview:
Qwen-72B is the newest LLM trained on 3 trillion tokens, published by the Qwen Team in collaboration with the Alibaba Group
Multilingual: performs extremely well on Chinese & English with vocabulary range of 150K tokens
Specs: maintain a context window of 32K with system prompt capability (eg, role playing, language style transfer, task setting, and behavior setting)
Evaluation: Top scorer in Chinese and English downstream evaluation tasks (including commonsense, reasoning, code, mathematics)
Qwen-1.8B:
New smaller model released along Qwen-72B that maintains essential functionalities and efficiency
2K-length text content with just 3GB of GPU memory

benchmark of all Qwen models
💻 Extracting Training Data from ChatGPT
Milad Nasr, Nicholas Carlini, Jon Hayase, Matthew Jagielski, A. Feder Cooper, Daphne Ippolito, Christopher A. Choquette-Choo, Eric Wallace, Florian Tramèr, Katherine Lee

prompt injection attack by spamming
Overview
Extract megabytes of ChatGPT’s training data for about two hundred dollars, even on well aligned models. Can potentially get gigabytes with even more money
Method: Asking ChatGPT to repeat a word indefinitely which led the model to break from its usual aligned behavior to its pre-training data. This then causes it to escape its fine-tuning alignment procedure and regurgitate its pre-training data
Exploitation findings:
The exploit does not work every time it's run
Approximately 3% of the text emitted following the repeated token was identified as memorized
The exploit's effectiveness may have changed since OpenAI was informed about it

previous attack v.s. new “repeat the word“ attack
Evaluation: Although ChatGPT initially appeared to have minimal memorization due to its alignment, their new attack method caused it to emit training data three times more often than any other model tested

You can view the entire transcript here

repeating infinitely causes ChatGPT to escape its guidelines
Complexity: While models are getting better at understanding and generating human-like text, controlling what they learn and remember from their training data is still a complex issue, as ensuring that the model doesn't memorize any sensitive or specific information is extremely difficult from such a huge amount of data
Disclosure: The exploit was discovered in July, reported to OpenAI on August 30, and publicly released after a standard 90-day disclosure period, adhering to ethical standards
Conclusion: Test AI models both before and after alignment, as alignment can be fragile and conceal vulnerabilities
Industry News
📽️ Pika 1.0: Text-To-Video
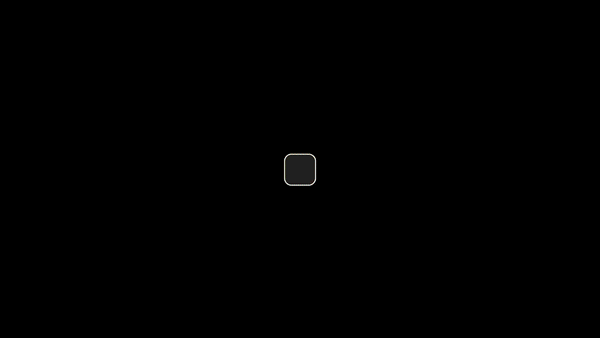
check the full trailer below
Overview
PikaLabs, launched six months ago as a startup with only a few researchers, they challenged big players like Runway in text-to-video generation, making it simple and accessible for everyone through Discord. It has quickly grown a community of half a million users because of it’s comparable quality to Runway’s Gen-2
Pika 1.0
The latest upgrade Pika 1.0 introduces an advanced AI video generation model capable of generating and editing videos in various styles, eg. 3D animation, anime, cartoon, and cinematic
Offers a variety of functions such as text-to-video, image-to-video, text+image to video, video inpainting, vidoe uncropping
You can find more information in the below tweet:
Introducing Pika 1.0, the idea-to-video platform that brings your creativity to life.
Create and edit your videos with AI.
Rolling out to new users on web and discord, starting today. Sign up at pika.art
— Pika (@pika_labs)
2:38 PM • Nov 28, 2023
💎 Millions of new materials discovered with deep learning
Amil Merchant and Ekin Dogus Cubuk

Overview
Groundbreaking Discovery: AI tool Graph Networks for Materials Exploration (GNoME) discovers 2.2 million new crystals, including 380,000 stable materials, by predicting their stability
Why It’s Revolutionary: Stable crystals are crucial for technologies like computer chips, batteries, and solar panels. Among the 380,000 stable materials, many are promising candidates for transformative technologies, including superconductors and advanced batteries
Methodology: GNoME uses graph neural networks and active learning to predict low-energy, stable materials, outperforming traditional approaches in accuracy and efficiency

GNoME’s architecture
Evaluations: 736 of these new structures have been experimentally created by external researchers and labs, validating GNoME's predictions
Data Availability: GNoME's findings are contributed to the Materials Project database, making them accessible to the research community for further exploration

New materials: Alkaline-Earth Diamond-Like optical material (Li4MgGe2S7) & a potential superconductor (Mo5GeB2)
Implications: GNoME's discovery represents an equivalent of 800 years of knowledge, showcasing AI's potential in material science

🚄 SDXL Turbo: A Real-Time Text-to-Image Generation Model
Axel Sauer, Dominik Lorenz, Andreas Blattmann, Robin Rombach

the image generation is in real-time
Overview:
Achieved SDXL SoTA speed performance through Adversarial Diffusion Distillation (ADD), reducing image generation steps from 50 to a single step, enhancing quality and efficiency

Adversarial Diffusion Distillation
Adversarial Diffusion Distillation
ADD integrates score distillation with adversarial loss, using existing large-scale image diffusion models as a teacher signal, so this combination maintains high fidelity in low-step (1-2 steps) image generation
The ADD-student model, functioning as a denoiser, processes diffused input images and optimizes two objectives:
Adversarial Loss: The model aims to deceive a discriminator that differentiates between generated and real images.
Distillation Loss: The model aims to match the denoised targets of a frozen DM (Diffusion Model) teacher
Layman’s Explanation
Imagine you're teaching a robot to draw. You show it some great drawings (these are generated by SDXL) and tell it, "Try to draw like these." This process is called score distillation. Then, you add a twist: the robot has to fool an art critic (the "discriminator") who can tell if a drawing was made by a robot or a human. This challenge is the adversarial loss similar in GANs.
The robot starts with a blurry sketch (the diffused input image) and tries to clean it up (denoising) to make it look like the examples you showed (matching the denoised targets of a frozen diffusion model teacher)
So with the new method called ADD, the robot can make a pretty good drawing in just one or two tries (steps), faster than other robot artists that take many tries.
Evaluation
ADD surpasses existing few-step methods, including GANs and Latent Consistency Models, in a single step. It achieves the performance of leading diffusion models like SDXL in just four steps
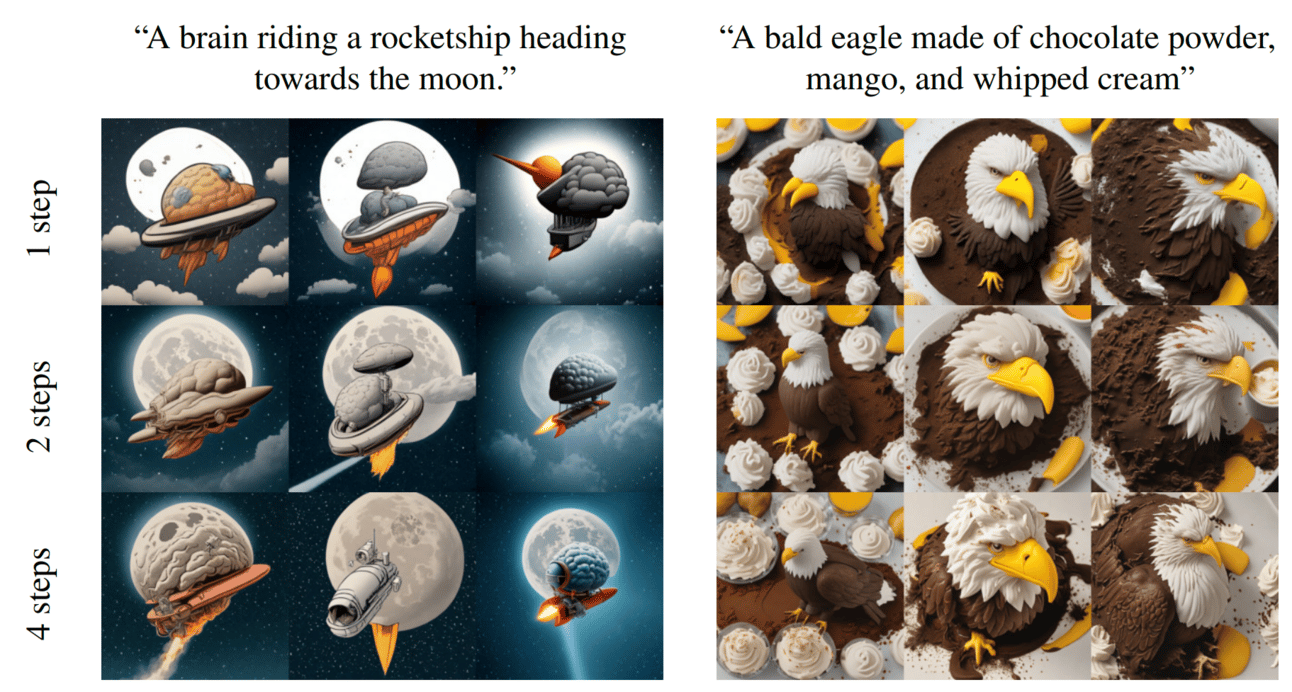
Qualitative effect of sampling steps

preference rating for image quality & prompt alignment
Access: You can use the real-time image generation with SDXL Turbo for free now on Clipdrop
that’s a wrap for this issue!
THANK YOU
Support me on Patreon ❤️
Want to promote your service, website or product? Reach out at [email protected]
Reply